Machine Learning Accuracy Metrics
Blog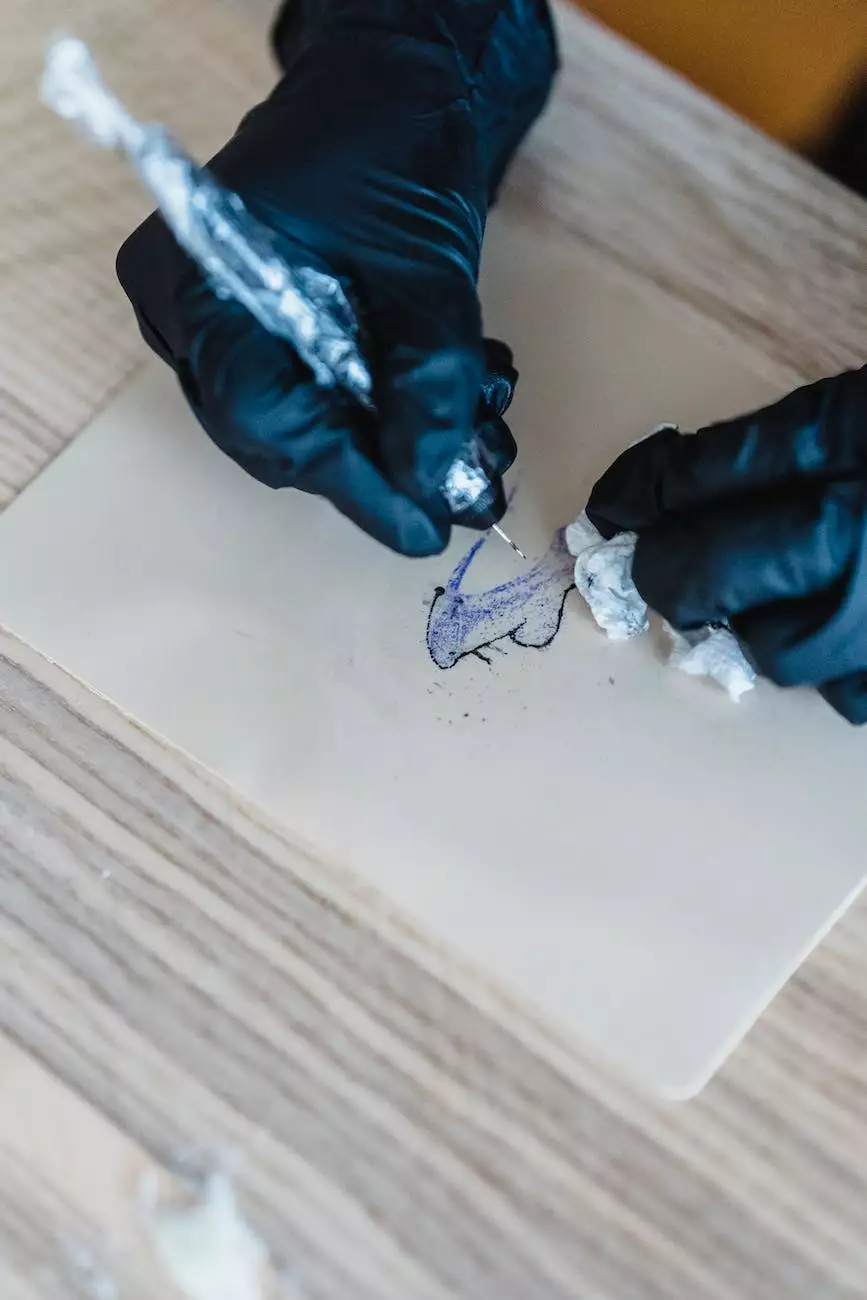
At Newark SEO Experts, we understand the importance of accurate and reliable machine learning models. In today's digital age, businesses heavily rely on data-driven decision making, and machine learning plays a crucial role in providing valuable insights and predictions. However, it is essential to evaluate the accuracy of these models to ensure that the results obtained are trustworthy and align with your business objectives.
Understanding Accuracy Metrics
Accuracy metrics are used to measure the performance of machine learning algorithms and models. They help in quantifying the accuracy and reliability of predictions made by these models, ultimately determining their effectiveness in solving real-world problems.
1. Accuracy
Accuracy is a commonly used metric to evaluate the performance of classification models. It measures the percentage of correctly predicted instances out of the total instances in the dataset. A higher accuracy score indicates a more reliable model, but it is crucial to consider other metrics as well.
2. Precision
Precision measures the proportion of true positive predictions out of the total predicted positives. It indicates how well the model correctly identifies positive instances. Precision is significant in scenarios where false positives can have severe consequences or when the cost of false positives is high.
3. Recall
Recall, also known as the true positive rate or sensitivity, measures the proportion of true positive predictions out of the total actual positives. It indicates the ability of the model to correctly identify positive instances. Recall is essential in scenarios where false negatives can have significant consequences or when the cost of missing positive instances is high.
4. F1 Score
The F1 score is the harmonic mean of precision and recall. It provides a balanced evaluation of the model's overall performance by considering both precision and recall. F1 score is useful when there is an imbalance between positive and negative instances in the dataset.
Evaluating Accuracy Metrics
Choosing the right accuracy metric depends on the specific requirements of your business and the nature of the problem you are trying to solve. To effectively evaluate machine learning models, it is crucial to consider the context and potential consequences of false positives and false negatives. Newark SEO Experts specializes in helping businesses navigate the complexities of accuracy metrics to ensure reliable and actionable insights.
Improving Accuracy Metrics
Maximizing the accuracy of machine learning models is a continuous process that requires expertise and iterative improvements. Newark SEO Experts offers cutting-edge solutions and strategies to improve accuracy metrics, ensuring a competitive advantage in the digital landscape.
Conclusion
Machine learning accuracy metrics play a fundamental role in assessing the performance of models and their relevance to business objectives. Newark SEO Experts understands the intricacies of accuracy metrics and offers tailored solutions to help businesses achieve accurate predictions and data-driven decision making. Partner with us to unlock the true potential of machine learning and propel your business towards success in the ever-evolving digital marketplace.