Mastering Machine Learning Data Labeling: The Key to Effective AI Solutions
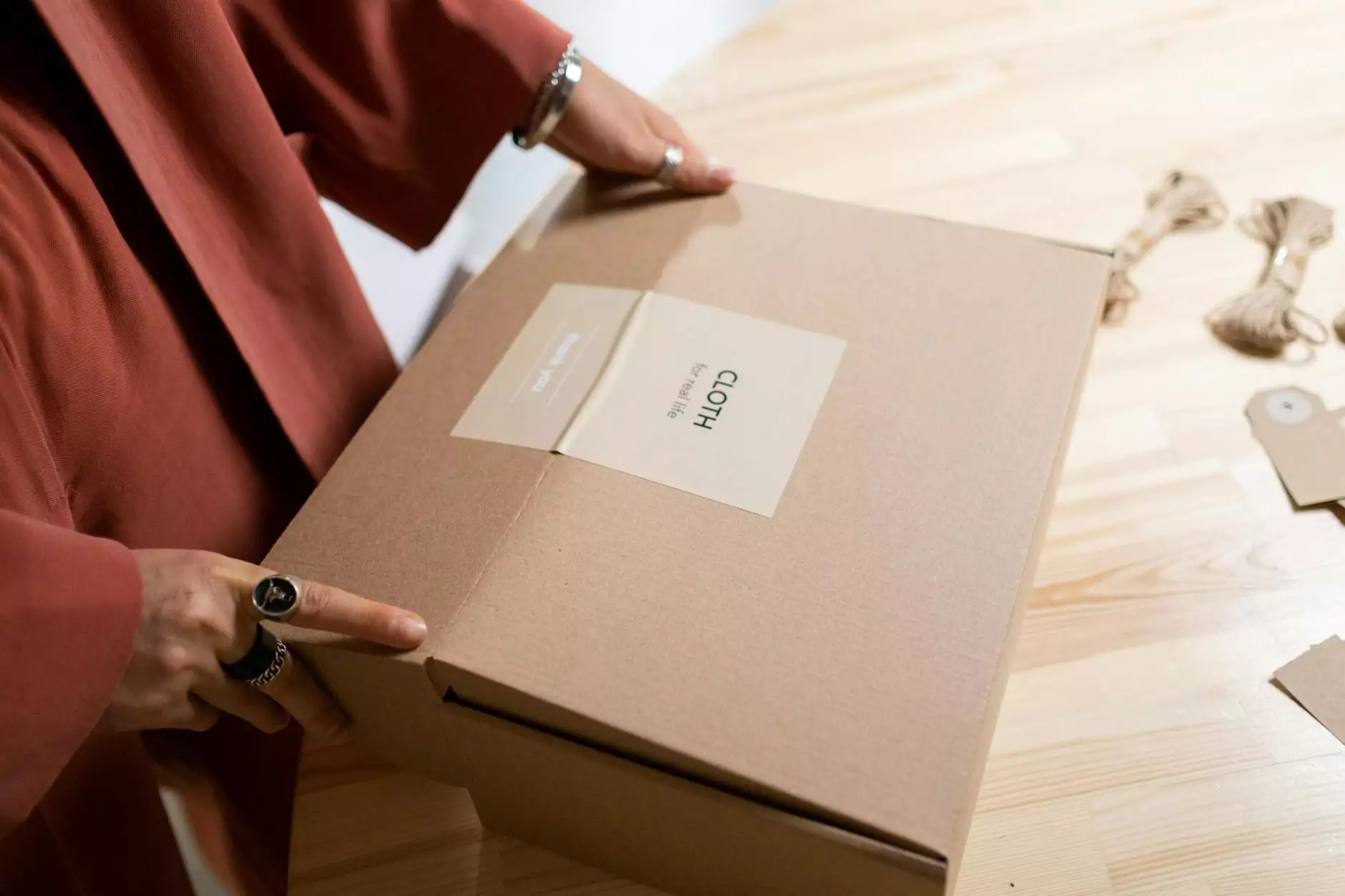
In today's rapidly evolving technological landscape, machine learning data labeling has emerged as a critical component for developing robust artificial intelligence applications. This article delves into the significance of data labeling, explores various methods and tools, and highlights how businesses can leverage these strategies through innovative platforms like Keylabs.ai.
What is Machine Learning Data Labeling?
Machine learning data labeling is the process of annotating data to create a training dataset for machine learning algorithms. It is essential because machine learning models learn from examples, and these examples must be accurately labeled to ensure the model understands the underlying patterns. Proper labeling allows algorithms to make predictions based on new, unseen data.
The Importance of Data Annotation in Machine Learning
The role of data annotation cannot be overstated. Without high-quality labeled data, machine learning models cannot perform effectively. Here are some reasons why data labeling is crucial:
- Accuracy: High-quality labeled data leads to more accurate models, reducing errors and increasing reliability.
- Performance: Well-labeled datasets enhance the performance of machine learning algorithms by providing clear guidance and context.
- Transfer Learning: Labeled data can be reused across different projects, promoting efficiency and reducing costs.
- Scalability: As businesses grow, their datasets expand. Proper labeling ensures that larger datasets remain manageable and useful.
Types of Data That Require Labeling
Data labeling can apply to various types of data. Understanding these types is essential for anyone involved in machine learning data labeling. The most common data types include:
- Textual Data: Involves labeling text data for applications such as sentiment analysis, entity recognition, and more.
- Image Data: Commonly used in computer vision projects, such as object detection and facial recognition.
- Audio Data: Involves labeling sound recordings for speech recognition and audio classification tasks.
- Video Data: Used in applications requiring action recognition or scene understanding that necessitates temporal labeling.
Methods of Data Labeling
Different methods can be employed for data labeling, each with its unique advantages and challenges:
- Manual Labeling: Involves human annotators manually labeling the data. While it can be time-consuming, it often results in high-quality data.
- Automated Labeling: Uses algorithms to label data quickly. While faster, it may lack the precision of manual labeling.
- Crowdsourcing: Involves distributing labeling tasks to a large number of people via platforms like Amazon Mechanical Turk. This method can be efficient but requires quality control measures.
- Active Learning: A machine learning technique where the model identifies data points it finds uncertain and requests labels for those specific instances.
Choosing the Right Data Annotation Tool
With the increasing need for machine learning data labeling, selecting the right data annotation tool has become vital. Businesses must consider several factors when choosing a data annotation platform, such as:
- User Interface: The tool should have an intuitive interface that allows labelers to work efficiently.
- Supported Data Types: Ensure the tool can handle the specific types of data relevant to your projects.
- Collaboration Features: The ability to team up on projects enhances productivity, especially for larger teams.
- Quality Control Mechanisms: Look for features that support quality assurance, like double-checking labels and audit trails.
- Integration Capabilities: The tool should seamlessly integrate with your existing workflows and machine learning frameworks.
Keylabs.ai: Your Ultimate Data Annotation Platform
One of the leading platforms in the realm of machine learning data labeling is Keylabs.ai. This advanced data annotation tool simplifies the process of preparing datasets for machine learning models, equipped with features designed to enhance efficiency and accuracy.
Features of Keylabs.ai
Keylabs.ai offers a wide range of features that cater to various data annotation needs:
- Multi-format Support: Whether you work with images, text, videos, or audio, Keylabs.ai can handle it all.
- User-Friendly Interface: The platform’s intuitive design ensures that both technical and non-technical users can navigate with ease.
- Collaboration Tools: Multiple users can work on the same project, with features to track progress and share feedback.
- Fast Turnaround: Automated processes speed up data labeling, allowing teams to scale efficiently.
- Quality Assurance: Advanced mechanisms ensure high accuracy and consistency in data labels.
Best Practices for Data Labeling
To maximize the quality and efficiency of machine learning data labeling, businesses should adhere to best practices, including:
- Define Clear Guidelines: Provide annotators with clear instructions and examples to ensure consistent labeling.
- Use a Small Sample First: Test labeling guidelines on a small dataset to identify challenges and refine processes before scaling.
- Incorporate Feedback Loops: Regularly review the labeled data and provide feedback to improve labeling quality.
- Implement Quality Checks: Use a combination of automated and manual reviews to maintain high standards.
- Stay Updated: As machine learning techniques evolve, keep your labeling methods and tools updated to align with best practices.
Conclusion: The Future of Machine Learning Data Labeling
The importance of machine learning data labeling cannot be overstated as it is directly tied to the success of AI applications. By investing in robust data annotation tools and following best practices, businesses can unlock the full potential of their data, leading to more effective AI solutions.
With platforms like Keylabs.ai providing tailored solutions for data annotation, organizations can enhance their machine learning capabilities, streamline workflows, and ultimately gain competitive advantages in their respective industries. The future of AI hinges on the quality of data that fuels it, and effective labeling processes are the key to harnessing that data efficiently.
Call to Action
Ready to elevate your AI projects with high-quality data labeling? Discover how Keylabs.ai can transform your data annotation process and help you unleash the power of machine learning in your business.