3 Ways to Analyze the Results of a Supervised Machine Learning Model
Blog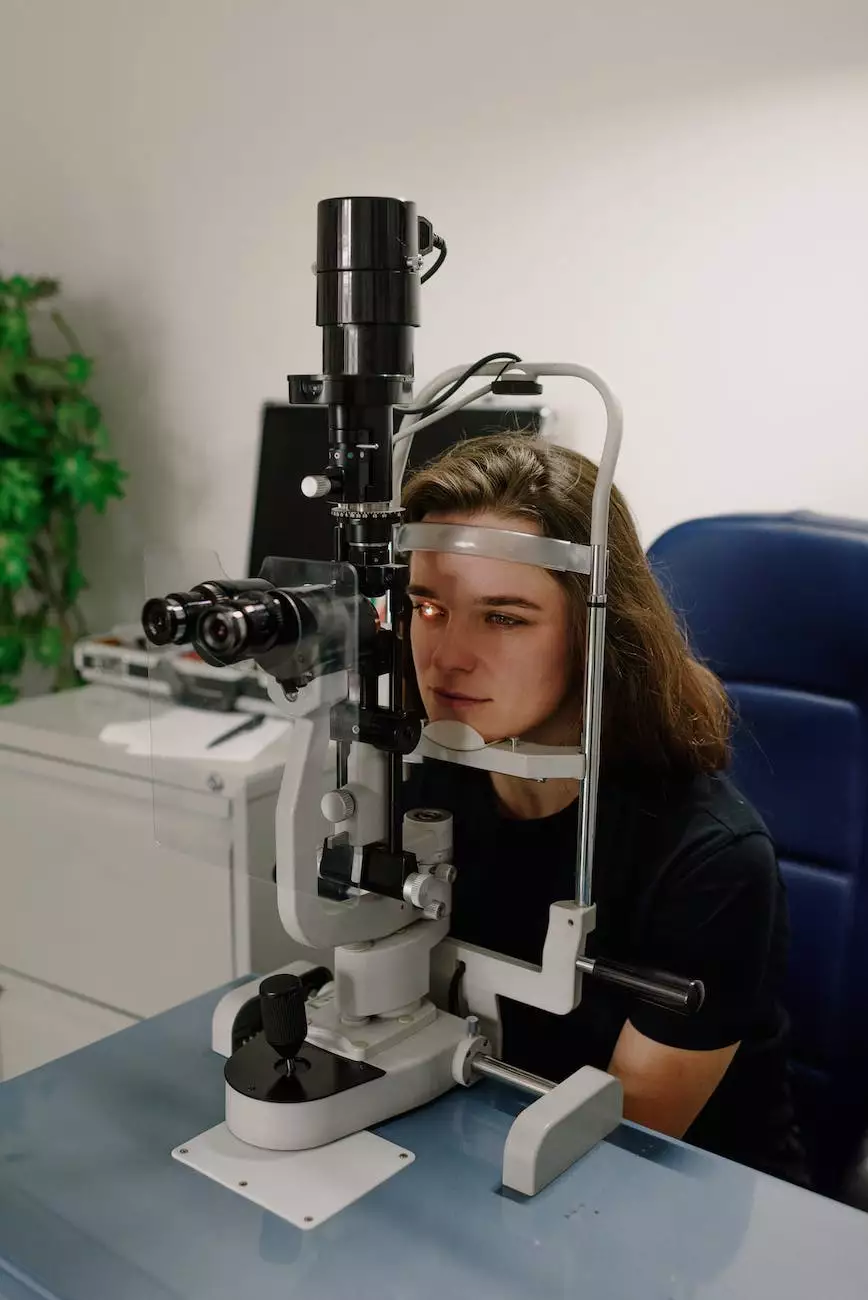
Introduction
Welcome to Newark SEO Experts, a leading digital marketing agency in the business and consumer services industry. In today's fast-paced digital world, businesses are increasingly relying on supervised machine learning models to gain valuable insights and make data-driven decisions. However, analyzing the results of such models can be a complex task.
Understanding Supervised Machine Learning
Before delving into the ways to analyze the results of a supervised machine learning model, it's important to understand the concept itself. Supervised machine learning is a branch of artificial intelligence that involves training a model on a labeled dataset, where input features are mapped to known output labels. The trained model is then used to predict labels for new, unseen data.
1. Accuracy and Performance Metrics
One way to analyze the results of a supervised machine learning model is by assessing its accuracy and performance metrics. Accuracy refers to how well the model predicts the correct output labels. Performance metrics, such as precision, recall, and F1-score, provide further insights into the model's ability to correctly classify different classes within the dataset.
By calculating these metrics, businesses can evaluate the effectiveness of the machine learning model in solving the specific problem it was trained for. Newark SEO Experts employs advanced techniques to measure accuracy and performance metrics, ensuring our clients receive reliable and actionable results.
2. Feature Importance Analysis
Understanding the importance of different features in the supervised machine learning model is crucial to gaining deeper insights into the underlying patterns and relationships within the dataset. Feature importance analysis helps identify the most influential factors that contribute to the model's predictions.
At Newark SEO Experts, our experienced data scientists utilize various feature selection techniques, such as recursive feature elimination and permutation importance, to determine the significance of each input feature. By focusing on the most important features, businesses can refine their strategies and make informed decisions based on the extracted insights.
3. Error Analysis and Model Fine-Tuning
Error analysis plays a vital role in improving the performance of a supervised machine learning model. By analyzing the mistakes or misclassifications made by the model, businesses can identify common patterns and sources of errors.
Newark SEO Experts conducts thorough error analysis by examining false positives, false negatives, and any biases present in the model's predictions. This analysis helps in identifying potential areas for model improvement and fine-tuning. Our team of experts implements corrective measures to reduce errors and enhance the overall performance of the machine learning model.
Conclusion
In the ever-evolving field of digital marketing, effective analysis of supervised machine learning model results is crucial. Newark SEO Experts, a trusted name in the industry, provides businesses with comprehensive insights and strategies to make the most out of their machine learning investments.
By considering accuracy and performance metrics, conducting feature importance analysis, and performing error analysis for model fine-tuning, businesses can optimize their decision-making processes and stay ahead of the competition.
Contact Newark SEO Experts today to leverage the power of supervised machine learning and revolutionize your digital marketing efforts!